This blog is the first of a series on AI business challenges, written by Xomnia’s analytics translators.
Have you ever played a game without fully understanding how to win? It’s frustrating. In part because you probably end up losing, but also because you’re never quite in control — you’re “being played”. Most games, even simple ones like tic-tac-toe, require a strategy to win. With different strategies competing, a game can be analyzed afterwards to understand how a certain strategy proved to be superior. This helps you win the next game.
Companies do the same: the core of their business strategy is a desired state (“winning”, in short) and a plan to get there. It involves prioritizing some goals over others and investing resources accordingly. With artificial intelligence (AI), some strategizing can be automated. For example, automated strategies (algorithms) can be used in trading to deal with super-human reaction times or processing capacity. Some cutting-edge AI programs don’t even need to be told the rules, and plan a winning strategy in a game of chess or Go along the way.
AI FOMO
AI, however, isn’t a cure-all. In complex environments, like a company creating products for a competitive international B2C market, there currently aren’t any real ways to automate business strategies. Rather, AI offers new ways of interacting with customers, designing products and services, and streamlining work. As such, it’s wise to at least consider them when creating a business strategy. But how to do that when catching up on AI news (deepfakes, bots writing poetry, autonomous vehicles) can sometimes feel like reading a sci-fi novel? Executives longing to take their company’s data capabilities one step further may experience AI FOMO when learning about all these state-of-the-art use cases and success stories.
With this backdrop, how do you get a complete overview of the AI use cases out there, decide which of these may be relevant, and then determine which ones actually add value to your company? Deciding rashly may lead to wasted time, energy and money — either because the benefits of a certain solution do not justify the investment, or because other use cases might be more bang for your buck. However, staying in AI-limbo for too long may result in missed opportunities and competitors gaining market advantage. You feel the urgency, but where do you start?
Download Xomnia's Way of Working whitepaper
How to determine the value of AI
Figuring out how to identify AI opportunities suited to a company’s situation can be quite the puzzle. A puzzle with lots of pieces and many different configurations depending on the context. Luckily, even the toughest puzzles can be solved with the right tools and a well-structured, analytical approach.
Let’s begin with some definitions, as the AI elements in a business strategy are sometimes separately referred to as “AI strategy”. The concept remains the same: an AI strategy, like a business strategy, is a plan to achieve a desired state, usually centered around adding value to a company. A strategy is necessary, because employees leave; teams are disbanded; new people join teams with different perspectives; business goals, products and focuses change over time too. Change is the only constant, which also explains why you need to evaluate your AI strategy frequently.
While this might sound logical, it doesn’t yet explain the “so what” of AI. Understanding and answering this “so what” is important because it ensures that data science & engineering teams have clear business goals (prevent hobby projects), and business stakeholders understand the possibilities of AI (prevent unrealistic expectations or missed opportunities). This alignment is needed to make sure data projects produce real, timely, and human-centered benefits — something that’s not as straightforward as it sounds (especially in large, complex organizations).
Three questions need to be clearly addressed when defining an AI strategy:
- WHAT are the AI opportunities for our company?
- WHY should our company chase these AI opportunities?
- HOW should our company chase these AI opportunities?
To answer questions 1 and 2, Xomnia developed an AI value proposition method (further discussed in this blog). To answer question 3, we developed an organizational assessment and roadmap method (to be discussed in a future blog). Collectively, the answers to these three questions form a natural starting point for a company’s AI journey towards business value; a shared story that’s essential for inspiring, guiding and connecting (self-managing) data product teams.
Back to step 1: Xomnia’s AI value proposition method involves three comprehensive activities for translating business challenges into AI solutions in a matter of weeks:
- Discovery workshop:
Like Bernard Marr wrote, to find AI opportunities in your business, you should (for now) forget the technology behind it. Start with the identification and ranking of pains and gains, and map these to business processes and company strategy (Vision, Mission, measurable goals / OKRs) using preparatory interviews, predefined exercises and canvases. - Use case definition:
With the prioritized pains and gains, create problem statements for the ones deemed most urgent and important. Ideate a long-list of applicable data solutions (AI/ML, data science, data engineering or otherwise), and match these with the problem statements. Review with stakeholders. - Analysis & selection:
Gather relevant data sources and explore them; model the business cases with available volume/ financial/ product/ customer data; and make a selection of the most promising use cases based on estimated business benefits (increased revenue, reduced cost, prevented churn, improved compliance).
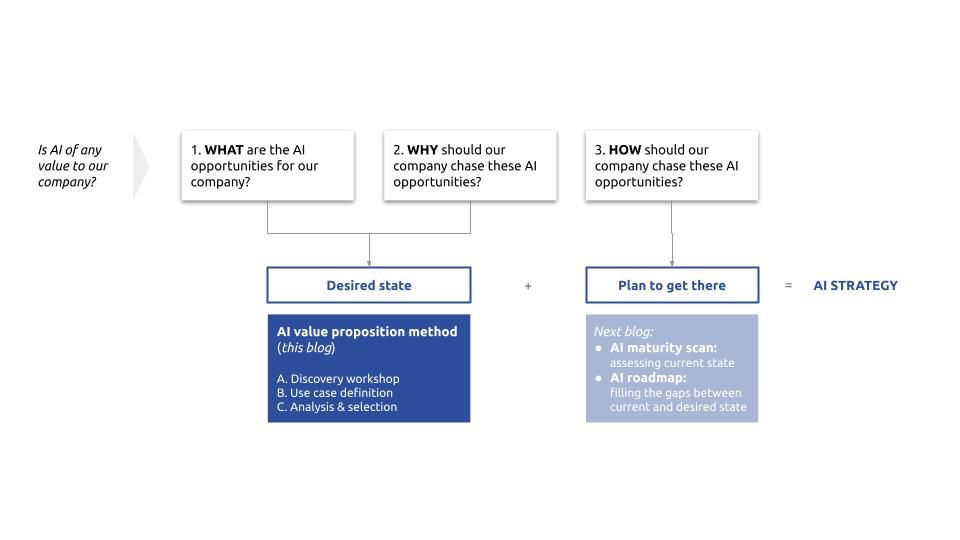
The AI value proposition method in practice: two client cases
Wereldhave, a Dutch commercial real estate company, partnered with Digital Sundai and Xomnia to determine which analytics projects best matched their LifeCentral strategy (“full service centers for better everyday life”) and digital journey. During the creation of Wereldhave’s analytics strategy, it was found that the ability to select the right tenants, optimize mall layouts, and properly determine rents greatly impacts revenues. To this end, tenant performance prediction, NPS driver analysis, tenant mix optimization, consumer spending pattern recognition, and new property valuation modelling use cases were selected for development.
Another client, a non-profit organization battling pollution, approached Xomnia to see if they could streamline their operations using data. It was discovered that camera footage from different locations was manually processed to determine where to focus pollution-cleaning efforts, which is prone to human error and inaccuracy. It was concluded that an object detection model could be used to automatically monitor footage at a scale, and thus make object classification and localization more accurate and consistent with limited human effort.
Download Xomnia's Way of Working whitepaper
—
Xomnia has a team of analytics translators (ATs) dedicated to assisting clients with the business aspects of AI challenges. They do so in several roles, such as AI Product Owner (product-focused), AI evangelist (adoption-focused), AI ethicist (responsibility-focused), or Strategic AI advisor (strategy-focused). In this last role, our ATs tackle the first of seven AI business challenges (creating a strong AI vision and plan aligned with the overall business strategy - topic of this blog). In our next AT blog, we’ll discuss how companies can grow their capabilities to enable development of the products on the AI roadmap.
Would you like to learn more or have a conversation about AI business challenges at your organization? Get in touch: info@xomnia.com.
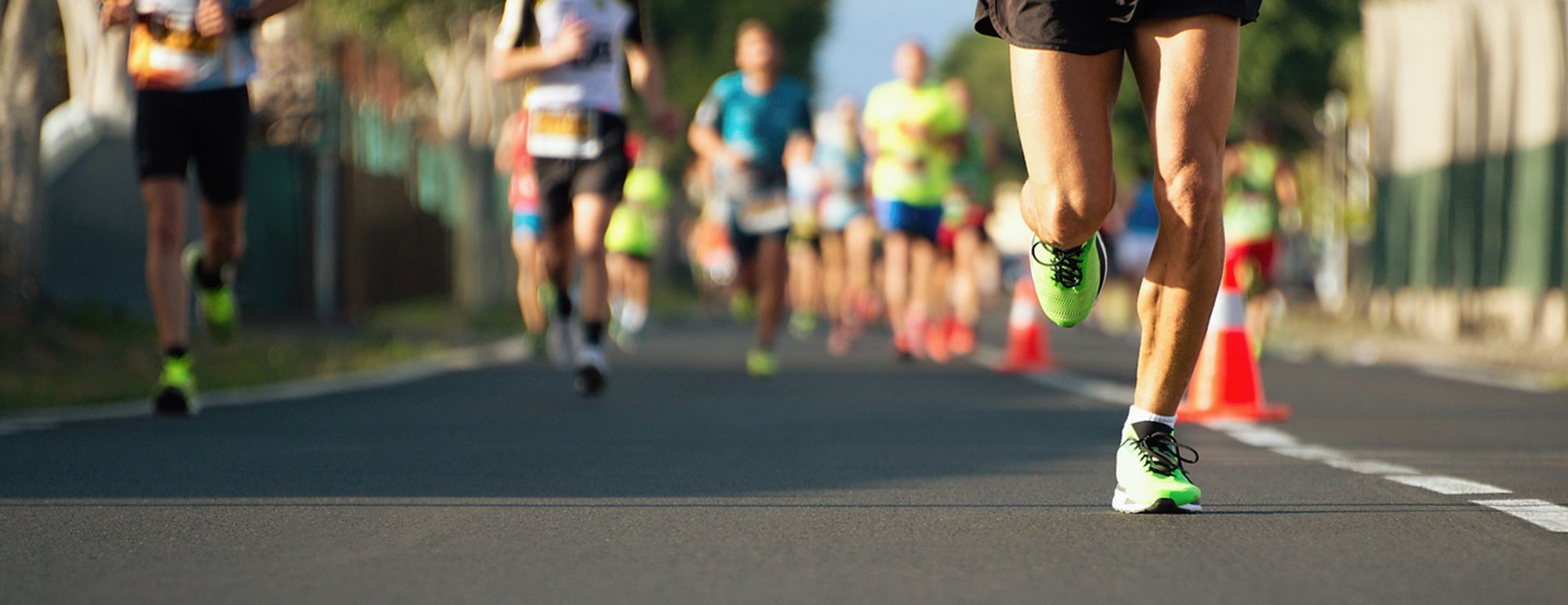