Enexis Netbeheer, one of the largest distributors of the electricity and gas grids in the Netherlands, has partnered with AI consultancy Xomnia to create 4 models to predict the areas that have a high likelihood of causing big disturbances on the electricity grid in the future.
The models will specifically give Enexis the necessary insights to accommodate the increasing number of households that install solar panels, heat pumps (hybrid or full electric), and charging points for electric vehicles (EVs). Enexis aims to utilize those predictions to future-proof its grid in the face of any overload that might result in disturbing its customers.
Our Machine Learning Engineer Martijn Beeks collaborated with a data scientist from Enexis to deliver the predictive models, which have passed the proof-of-concept phase and are currently in production.
"We invest millions of Euros annually on the electricity grid to meet consumers’ demand, but we’re facing challenges brought by a larger number of electric vehicles and technologies connected to the grid, in addition to shortages in human resources. Xomnia partners with us to address these challenges with their in-depth knowledge of AI and business logics." Thijs Kamperman from Enexis.
Challenge
As more households in the Netherlands move to installing heat pumps, solar panels, or charging points for electric vehicles, the grid in the Netherlands faces a lot of pressure to absorb or provide significant amounts of electric power over short periods of time.
Due to the limited capacity of all kinds of components in the grid, such as the cables or the transformers, Eenxis found itself facing a three-fold challenge: Ensuring that the network is ready for any disturbances and keeping electricity affordable all while dealing with the scarcity of technical personnel.
Enexis collaborated with Xomnia to produce predictive models to provide it with the necessary foresight to avert any future disturbances on the grid, and to make sure that its infrastructure is better prepared to accommodate sudden spikes in demand or supply of electricity on the grid.
Solution
The outcome of the project is 4 predictive machine learning models:
- Two models that predict the probability of installing 2 different types of heat pumps (hybrid or full electric) in a different geographies,
- a model that predicts the locations or households with a high probability of installing solar panels, and
- a model that is focused on predicting locations with a high likelihood of installing residential charging points for electric vehicles.
The machine learning models analyze data on household and neighborhood levels to understand relationships between the likelihood of installing one of the aforementioned technologies and factors like the household income, house price and building year, average household size in persons and square meters, percentage of owned houses in a neighborhood vs rented houses, the address density of a neighborhood, and others.
“Based on these models, we make predictions for connections that have not yet installed panels, heat pumps, and charging points. The outcome is the probability of getting one of them in the foreseeable future,” explained Machine Learning Engineer Martijn Beeks.
The models provide a probability of future adoptions of one of the aforementioned techniques and can be used to simulate the electricity grid to see which components cause congestion and should be replaced in the foreseeable future. The predictions are provided on a quarterly basis and will be stored in a database to be used for strategy setting and modeling.
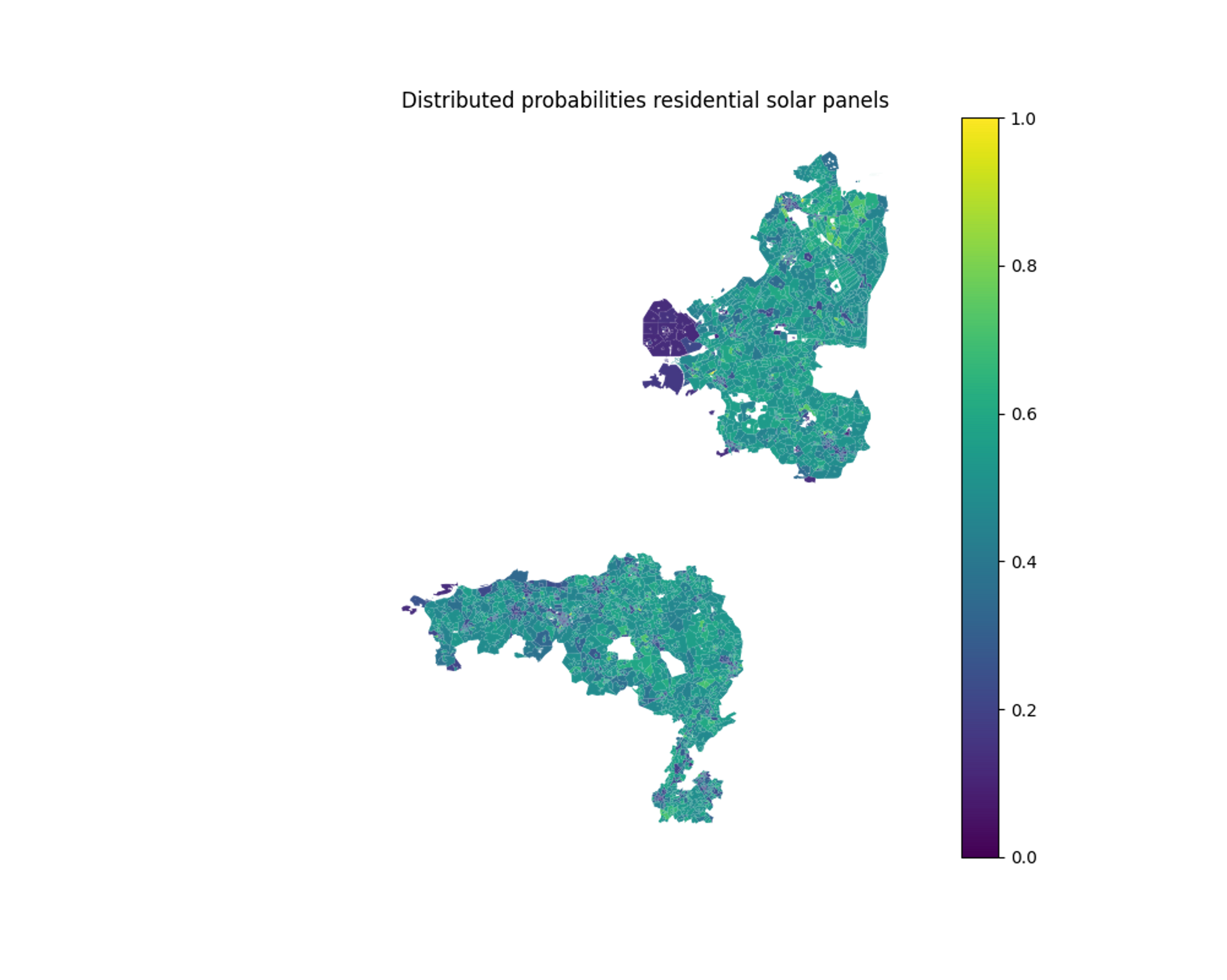
Impact
The models create insights that are expected to help Enexis in determining the best locations to enhance its infrastructure with accuracy and efficiency. This will help the grid operator better invest its resources while also ensuring a smooth and stable customer experience - by averting any interruptions due to overload on the electricity grid. Stay tuned for more updates about the project.